Day 1 :
- Computational Methodologies | Systems Biology | Metabolomics
Location: Athens
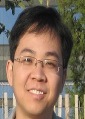
Chair
Tao Huang
Shanghai Institutes for Biological Sciences, China
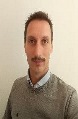
Co-Chair
Andrea Ragusa
University of Salento, Italy
Conference Series Ltd invites all the participants across the globe to attend 13th International Conference on Metabolomics and Systems Biology Hilton Zurich Airport Hohenbuehlstrasse 10, 8152, Opfikon, Switzerland.
Shanghai Institutes for Biological Sciences, China
University of Salento, Italy