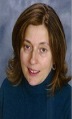
Alla Karnovsky
University of Michigan, USA
Title: Tools for data-driven network analysis of metabolomics data
Biography
Biography: Alla Karnovsky
Abstract
Metabolomics has established itself as a powerful platform to interrogate cellular biochemistry, identify novel biomarkers and provide insights into biochemical mechanisms of disease. As metabolomics data sets become increasingly large and complex, there is a growing need for data analysis and visualization tools to help interpret experimentally observed changes and put them into relevant biological context. A common approach to interpreting the results of metabolomics and lipidomics experiments is to map and visualize experimentally measured metabolites in the context of known biochemical pathways. Several tools for performing this type of analysis have been developed including our own tool Metscape. Some tools have adopted Functional Enrichment Testing methods developed for gene expression data for the analysis of metabolomics data. However, the scope of their application has been limited to known compounds from large, well-annotated pathways, which are often occupied by a small portion of the experimentally measured metabolome. An alternative to knowledge-based data analysis is to infer meaningful associations between metabolites/lipids from experimental data and build data-driven metabolic networks to help generate biological insights. We developed a new Differential Network Enrichment Analysis method (DNEA) that uses joint structural sparsity estimation to build partial correlation networks from the data (for two or more experimental conditions), performs consensus clustering to identify highly connected network components (subnetworks) and uses Network-based Gene Set Analysis (NetGSA) to identify the differentially enriched subnetworks. We will present the applications of DNEA for the analysis of metabolomics and lipidomics data and demonstrate that it allows identifying alterations in both network structure and expression levels of interacting biomolecules that impact disease phenotypes.