Day 1 :
Keynote Forum
Tao Huang
Shanghai Institutes for Biological Sciences, China
Keynote: Deciphering the mechanisms of complex diseases using network and machine learning approaches
Time : 09:40-10:25
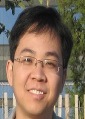
Biography:
Tao Huang has completed his Postdoctoral Fellowship in the Department of Genetics and Genomics Sciences, Icahn School of Medicine at Mount Sinai, New York City, USA. He is currently a Professor and the Director of Bioinformatics Core Facility at the Institute of Health Sciences, Shanghai Institutes for Biological Sciences, Chinese Academy of Sciences ( P R China). His research interests include bioinformatics, computational biology, systems genetics and big data research. He has published over 100 articles in peer-reviewed journals. His works have been cited for 3138 times with an h-index of 26 and an i10-index of 64. He has been an Independent Reviewer for 30 journals. He is the Editor of the book: “Computational Systems Biology - Methods and Protocols” published in Springer; Guest Editor for BBA Molecular Basis of Disease, BBA General Subjects, Artificial Intelligence in Medicine, BioMed Research International, Combinatorial Chemistry & High Throughput Screening and Computational and Mathematical Methods in Medicine.
Abstract:
The complex diseases, such as cancer, involves dysfunctions on multiple levels. There have been several methods to identify the dysfunctions on each level. On genomics level, GWAS (Genome-wide association study) can detect the disease phenotype associated SNPs (single nucleotide polymorphisms). But many GWAS identified SNPs locate in intergenic region and can't be annotated to specific genes. With the help of eQTL (expression quantitative trait loci) method, the downstream genes of the GWAS SNPs can be found. And based on the co-expression network, regulatory network, protein-protein interaction network, or even protein-chemical interaction network of these genes, the possible cascade of the signal transduction from the genetic perturbations can be discovered. By integrating all these omics data, we can establish a systems biology model of how the GWAS SNPs affect the expression of their direct target genes, how these direct targets affect secondary target genes, or proteins, or metabolites, and eventually cause the catastrophic pathological changes on network or pathway. With the comprehensive network, we can not only study the mechanism of one disease, but also the relationship of several traits. For example, schizophrenia and anti-tuberculosis drug-induced hepatotoxicity co-occurred frequently but they do not share common genes. We mapped their genes onto the network, the common drivers of these two diseases were revealed through two-way RWR analysis. Another example is that the lung cancer dysfunctions on several levels but how the different level dysfunctions connect are still unknown. We investigated the mutation, methylation, mRNA and microRNA expression difference between cancer and normal tissues using machine learning methods, then mapped the dysfunction of each level onto the comprehensive network. By connecting each other with shortest paths, the highly frequent shortest path genes that can cause the tumorigenesis on multiple levels were discovered. Overall, the network and machine learning approaches are effective to dissect the complex system and reveal the mechanisms of diseases.
Keynote Forum
Andrea Ragusa
University of Salento, Italy
Keynote: Metabolomics and lipidomic profile of clinical serum samples from patients with liver disease
Time : 10:45-11:30
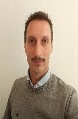
Biography:
Abstract:
Keynote Forum
Gerald C Hsu
eclaireMD, USA
Keynote: Relationship between metabolism and obesity along with type 2 diabetes and cardiovascular risk
Time : 11:30-12:15
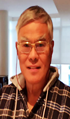
Biography:
Abstract:
- Computational Methodologies | Systems Biology | Metabolomics
Location: Athens
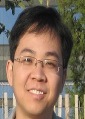
Chair
Tao Huang
Shanghai Institutes for Biological Sciences, China
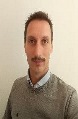
Co-Chair
Andrea Ragusa
University of Salento, Italy
Session Introduction
Abdullah Basoglu
Selcuk University, Turkey
Title: Effects of boron supplementation on peripartum dairy cows’ health: A metabolomic approach
Time : 12:15-12:45
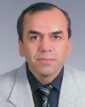
Biography:
Abstract:
Alla Karnovsky
University of Michigan, USA
Title: Tools for data-driven network analysis of metabolomics data
Time : 12:45 - 13:15
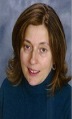
Biography:
Abstract:
Yan Ji
Shanghai Institutes for Biological Sciences, China
Title: SR splicing, splicing-ratio based splicing detection method
Time : 14:20-14:50
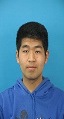
Biography:
Abstract:
Maroun Bou Sleiman
Swiss Federal Institute of Technology in Lausanne, Switzerland
Title: Enabling discovery through multi-omics and systems genetics
Time : 14:50-15:20
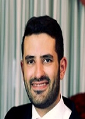
Biography:
Abstract:
Ryohei Eguchi
Nara Institute of Science and Technology, Japan
Title: Classification of biosynthesis pathways of alkaloids using graph convolutional neural networks
Time : 15:20-15:50
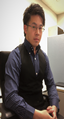
Biography:
Abstract:
- Metabolomics in Drug Discovery | Cancer Metabolomics | Transcriptomics and Proteomics
Location: Athens
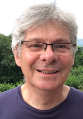
Chair
Philip J Jackson
University of Sheffield, UK

Co-Chair
Eloiza H Tajara
University of São Paulo, Brazil
Session Introduction
William Hidalgo
Industrial University of Santander, Colombia
Title: Transcriptomic and metabolic analysis of the plant hormones signalling pathways in the resistant “Calcutta 4†and susceptible “Williams†banana genotypes during the interaction with the pathogenic fungus Pseudocercospora fijiensis
Time : 12:35-13:05
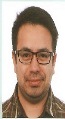
Biography:
Abstract:
Philip J Jackson
University of Sheffield, UK
Title: Proteomic adaptation in high and low light ecotypes of the marine picocyanobacterium Prochlorococcus marinus, an important global primary producer
Time : 13:05-13:35
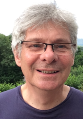
Biography:
Abstract:
Masahiro Onuma
Trisguide CO.,LTD, Japan
Title: Electronic water can reduce oxidative stress in cancer and diabetes patients for 3 weeks drinking
Time : 14:35-15:05
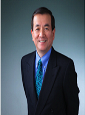
Biography:
Abstract:
Halina Malina
Axanton Technology GmbH, Switzerland
Title: Elimination the chemically modified proteins from the cell membranes prevents the cell degeneration
Time : 15:05-15:35

Biography:
Halina Malina pursued her PhD in antibiotic biosynthesis working at the Medical School of Lodz (Poland). She is a Chemical Engineer from Polytechnic of Lodz, Lodz. Since 1984, she worked at the Institute of Chemistry of Natural Substances (CNRS), with collaboration with Pasteur Institute of Paris (France). In 1990 she worked as project leader in CNRS University in Zurich. She discovered the IDO in the eye and the role of xanthurenic acid in the cataract development. She followed the research on xanthurenic acid-induced cell pathology at universities in Lausanne, and Bern and ETH. Her research on the chemical mechanism of the diseases with aging met a strong opposition of the academia healing the transgenic mice. All support for research was rejected in 2004. She continued on her own and established the technology curing the infection and the aging-associated pathology.
Abstract:
Vivek Kamath
Heal The World, India
Title: Case study of insulin dependent diabetes: Healing without medicines
Time : 15:35-16:05
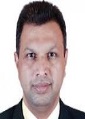
Biography:
Abstract:
As most of us are aware Type 2 diabetes can be controlled and cured completely with the diet, workouts (yoga), effective stress management and other healing methods. Testimonial of one of my patient aged 70 years, male suffering from diabetes from more than 12 years and took 3 insulin doses per day. He has completed Reiki Master Degree through some Reiki Master in India. He came to be with the problem that he is not able to feel the energy while doing his self healing. Moreover, he was suffering major health problems like (Insulin dependent diabetes for nearly 12 years, insomnia, constipation, stomach heaviness, etc.). I gave him Reiki Level 1 attunement a month back. On 25th July he has taken his random glucose reading. The random glucose level was 575 mg/dl. On July 27th I have given him Usui Reiki Level 2, 3 Attunement, Karuna Reiki Attunement and Mexican Healing attunement (Guru Poornima Day). Immediately after the attunement we checked his random glucose level it came down to 383 mg/Dl. After 1 Mexican Healing: On 28th July evening, I conducted Mexican healing training class to my patient along with others. I took his random glucose level before giving him Mexican healing and it was 344 mg/Dl. After 1 Mexican healing his glucose came down to 260 mg/Dl. All the people who attended the training class were astonished with the power of Mexican healing. To summarize in 3 days, using attunement (Reiki and Mexican attunement) and with 1 Mexican healing, his glucose level came down from 575 mg/dL to 260 mg/DL. What is the percentage in drop down? 54.8%. Is possible to bring this much of level down in 3 days without taking insulin? Please note that there is no change in his food/diet or workouts. Also note the difference between the readings after attunement and after healing. We are all made up of energy and it flows though the energy centers (chakra). We can’t necessarily see energy, but we can feel the energy. When our energy centers becomes weak, diseases related to corresponding energy centers will pop up. For Diabetes, there is energy center called “Solar Plexus” (Also called as Manipura Chakra). All diabetic patients will have low energy in their solar plexus energy. If you energize this energy center you will see the drastic reduction in your glucose level. You do not need to go for a pancreas transplant or tablets/insulin to treat your diabetes. It is all in the energy. Learn from your Reiki master or healers to energize all your weaker organs or energy centers.